0.Long Short Term Memory (LSTM)
LSTM希望神经网络能够「记住」或者「忘记」一些信息,从而有更好的表现。
当需要的信息和预测之间的时间间隔比较小的时候,RNN可以比较好的效果,如下图:
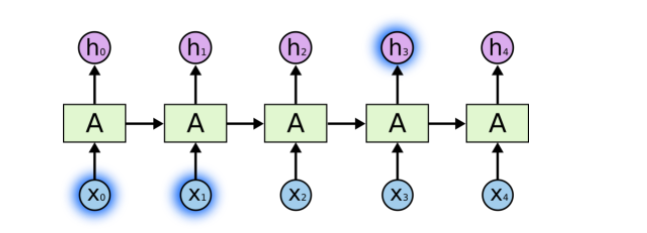
当需要的信息和预测之间的时间间隔比较大的时候,RNN的效果特别差, 如下图:
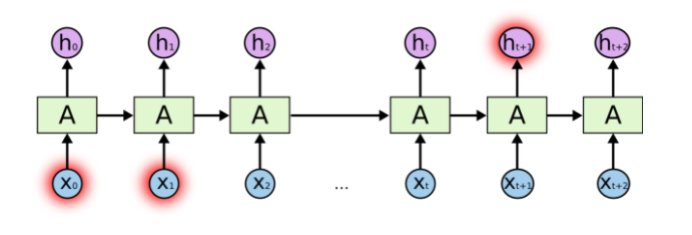
与此同时,当时间序列长度很长时,在梯度反向传播时,过多层数的梯度积累,会使得梯度爆炸和梯度消失的情况出现。
LSTM就是基于上述原因提出的,对于某个时间点之前的信息进行选择性记忆,然后再进行预测,所以LSTM相较于RNN添加了很多类似于人的遗忘门和决定门等。
1.LSTM Networks
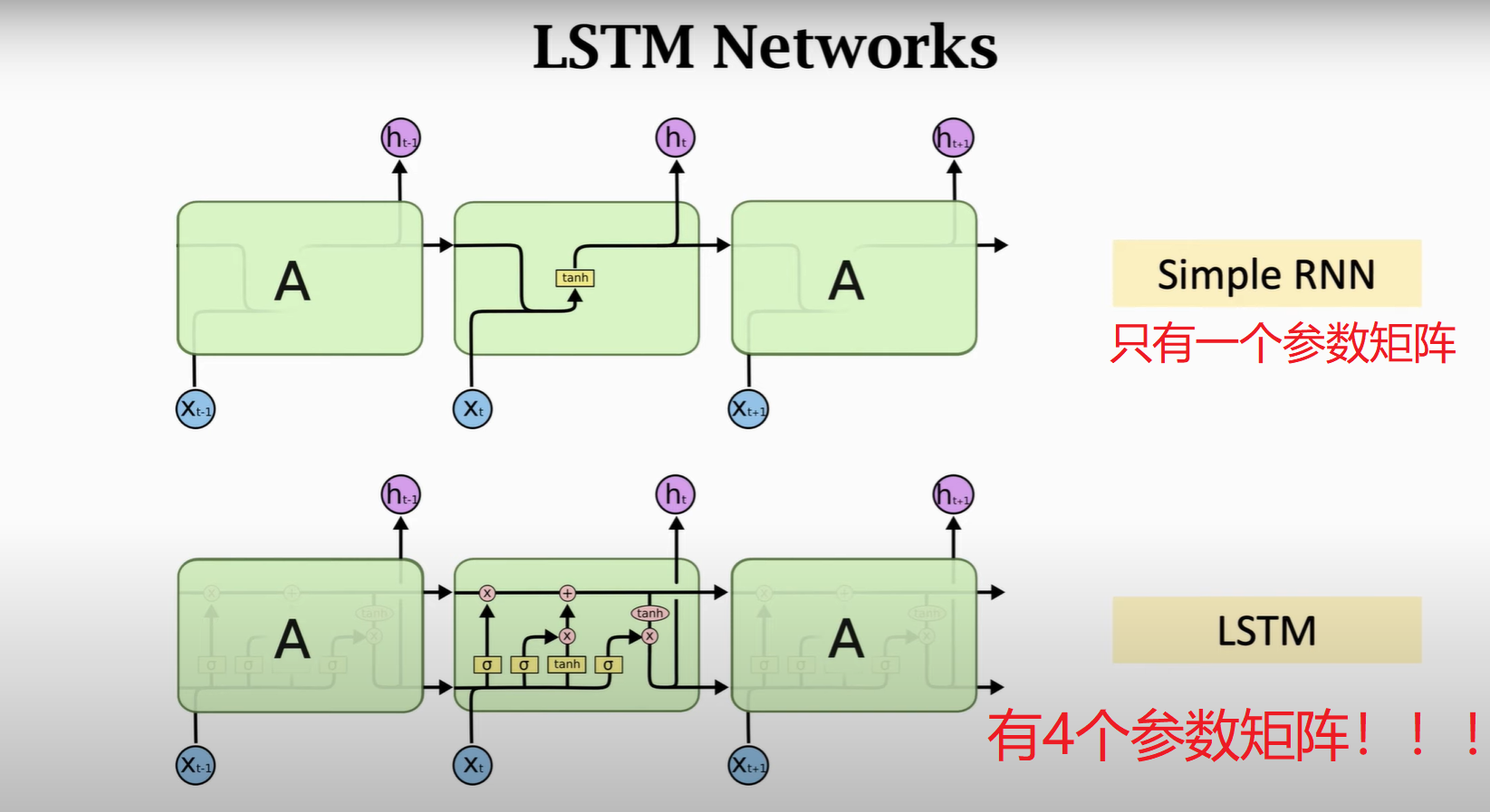
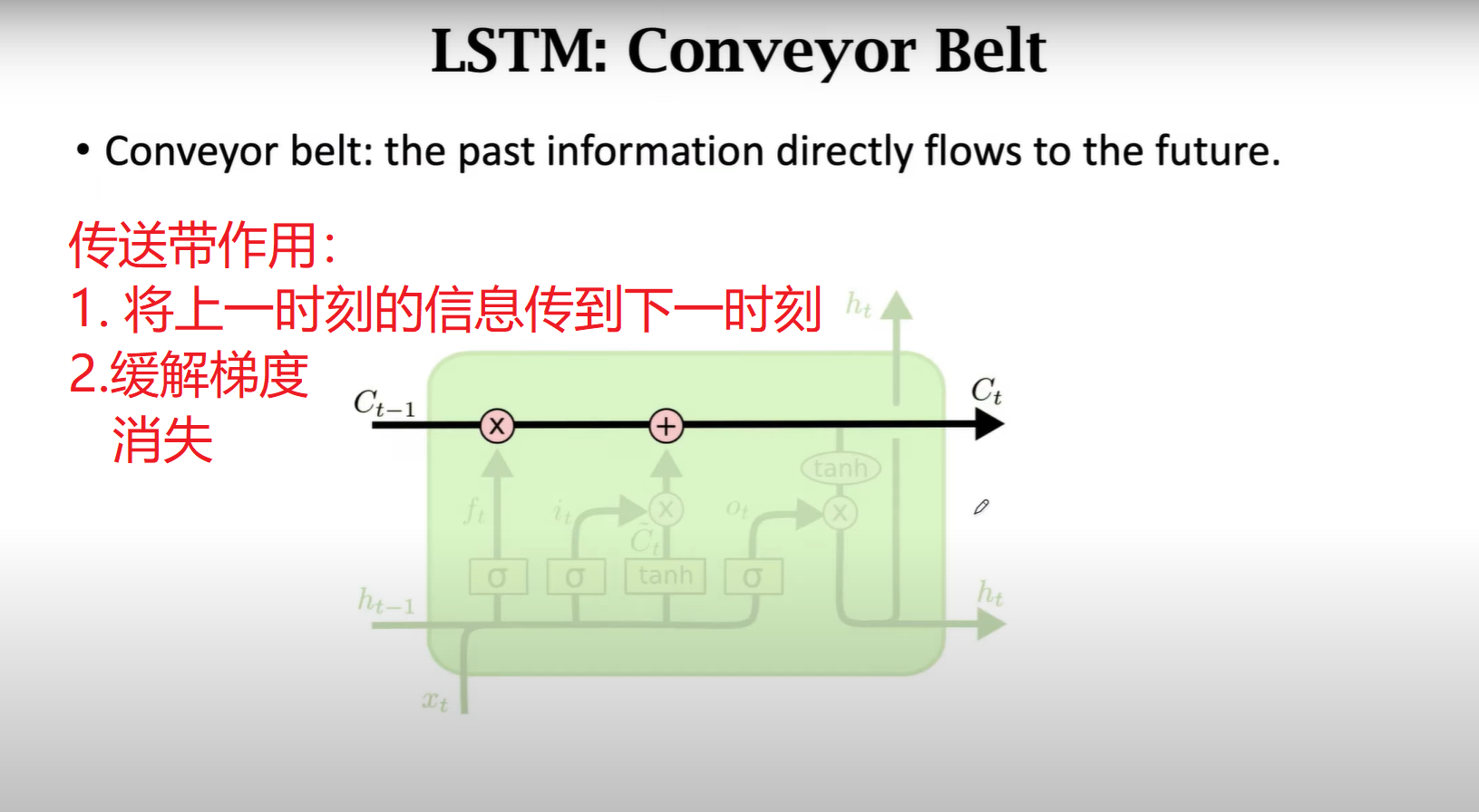
1.1 Forget Gate
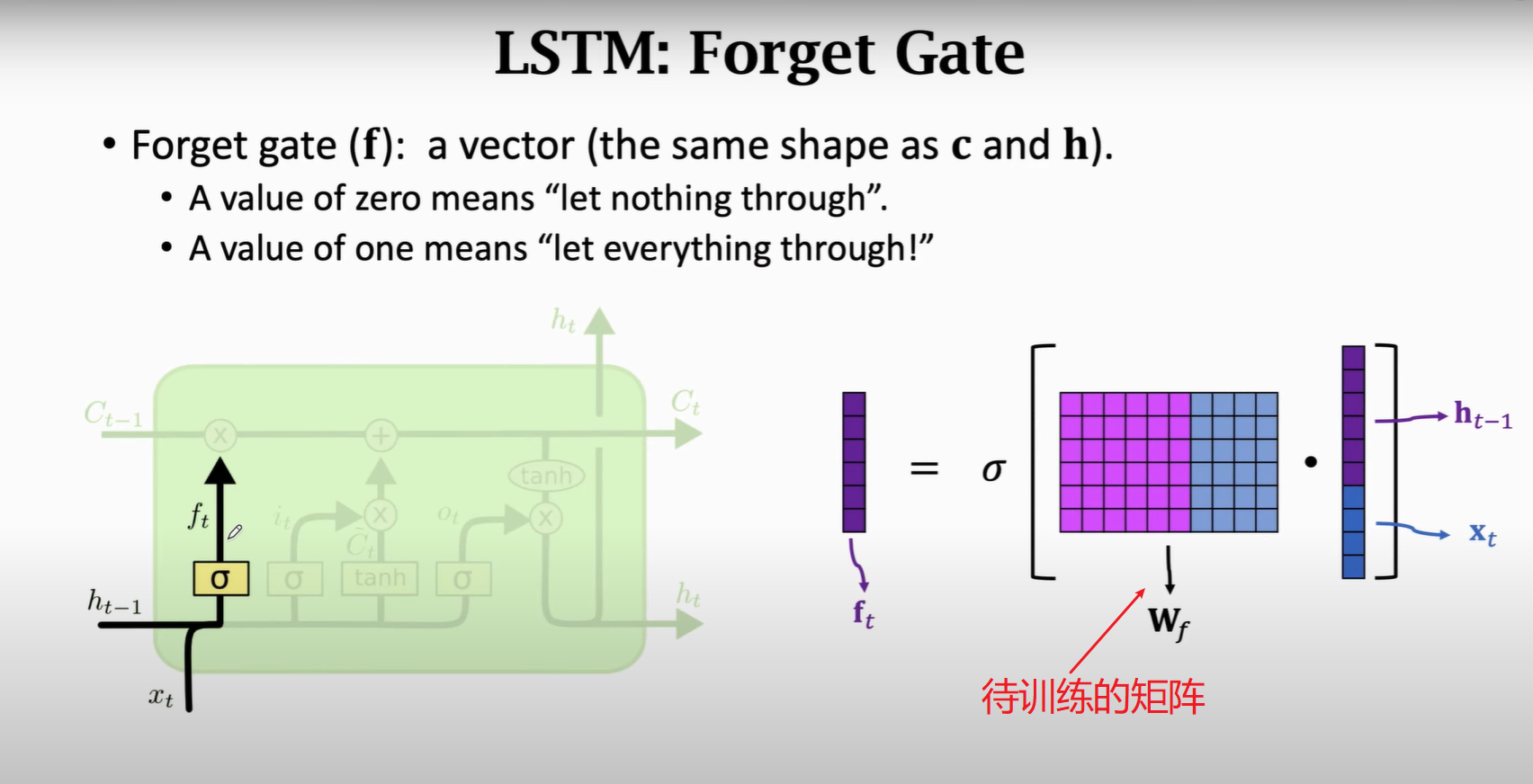

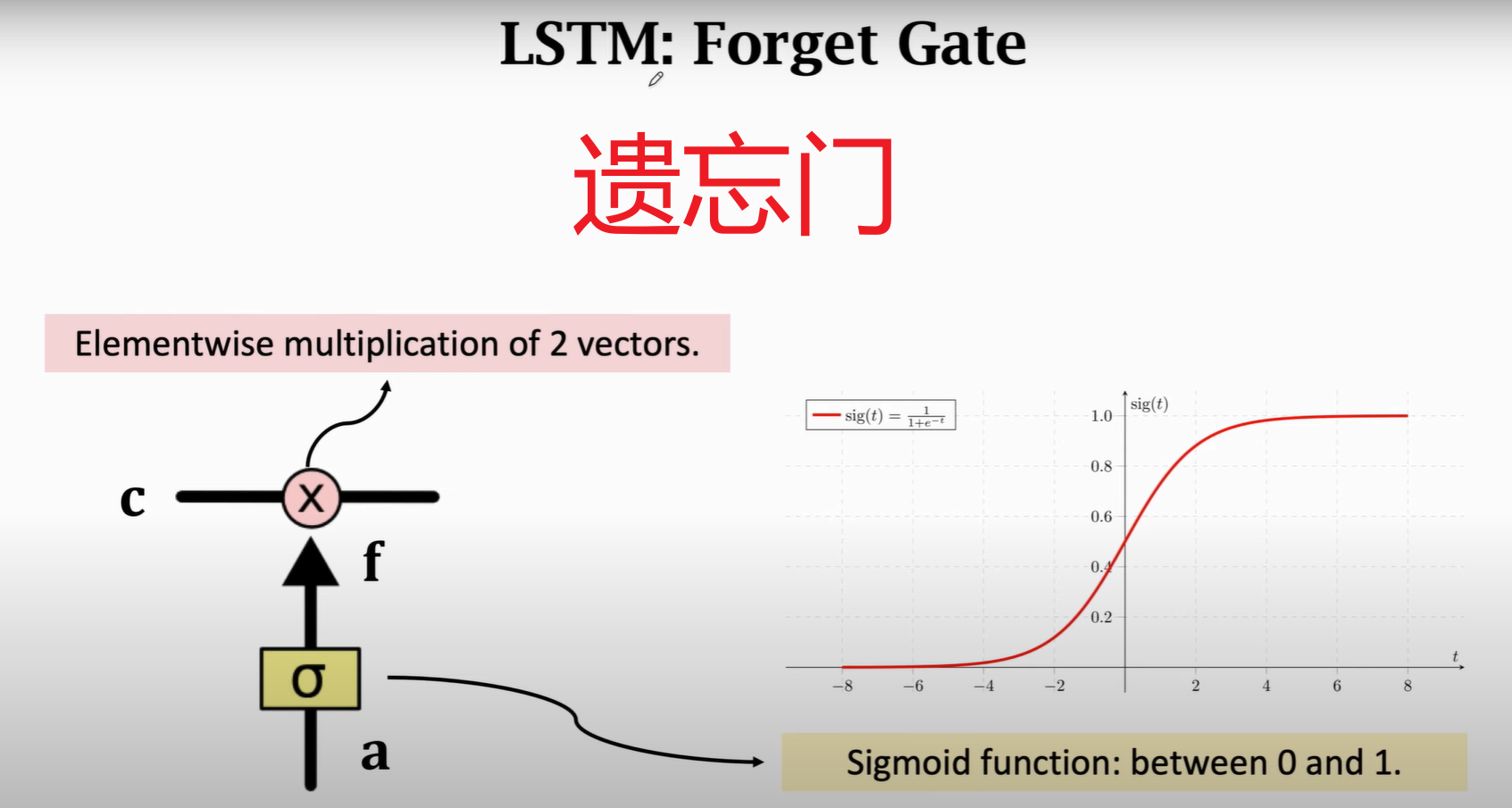
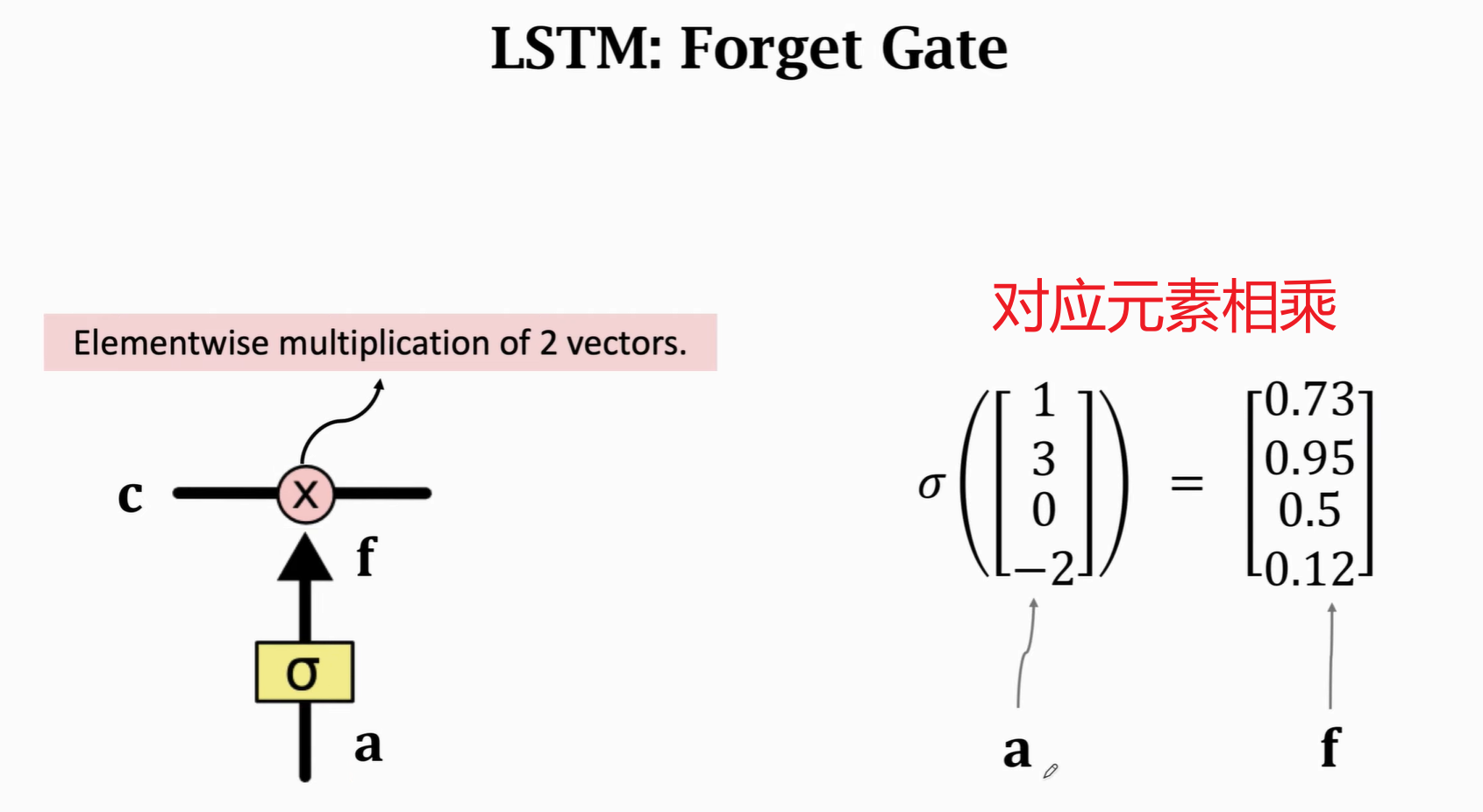
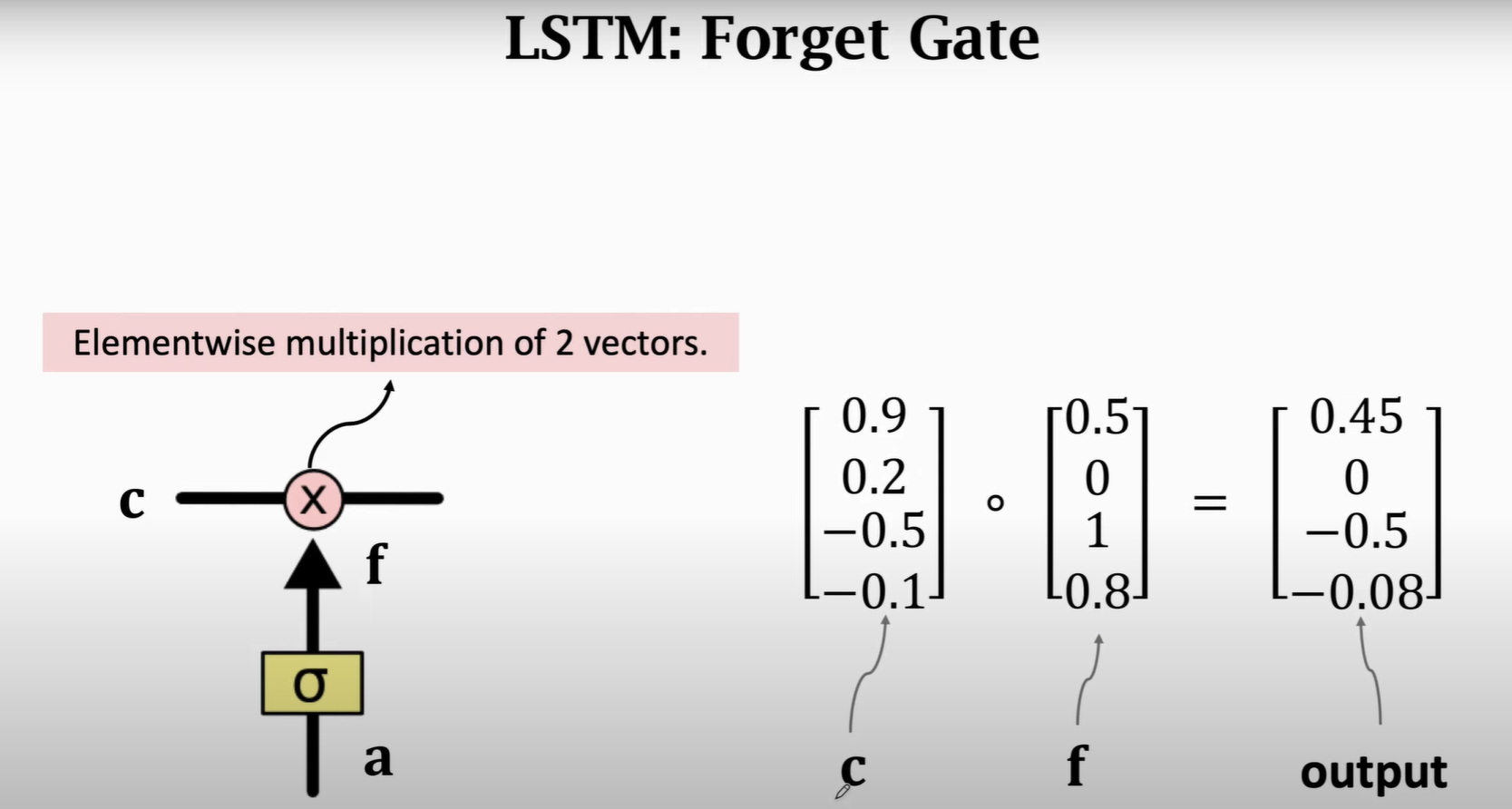
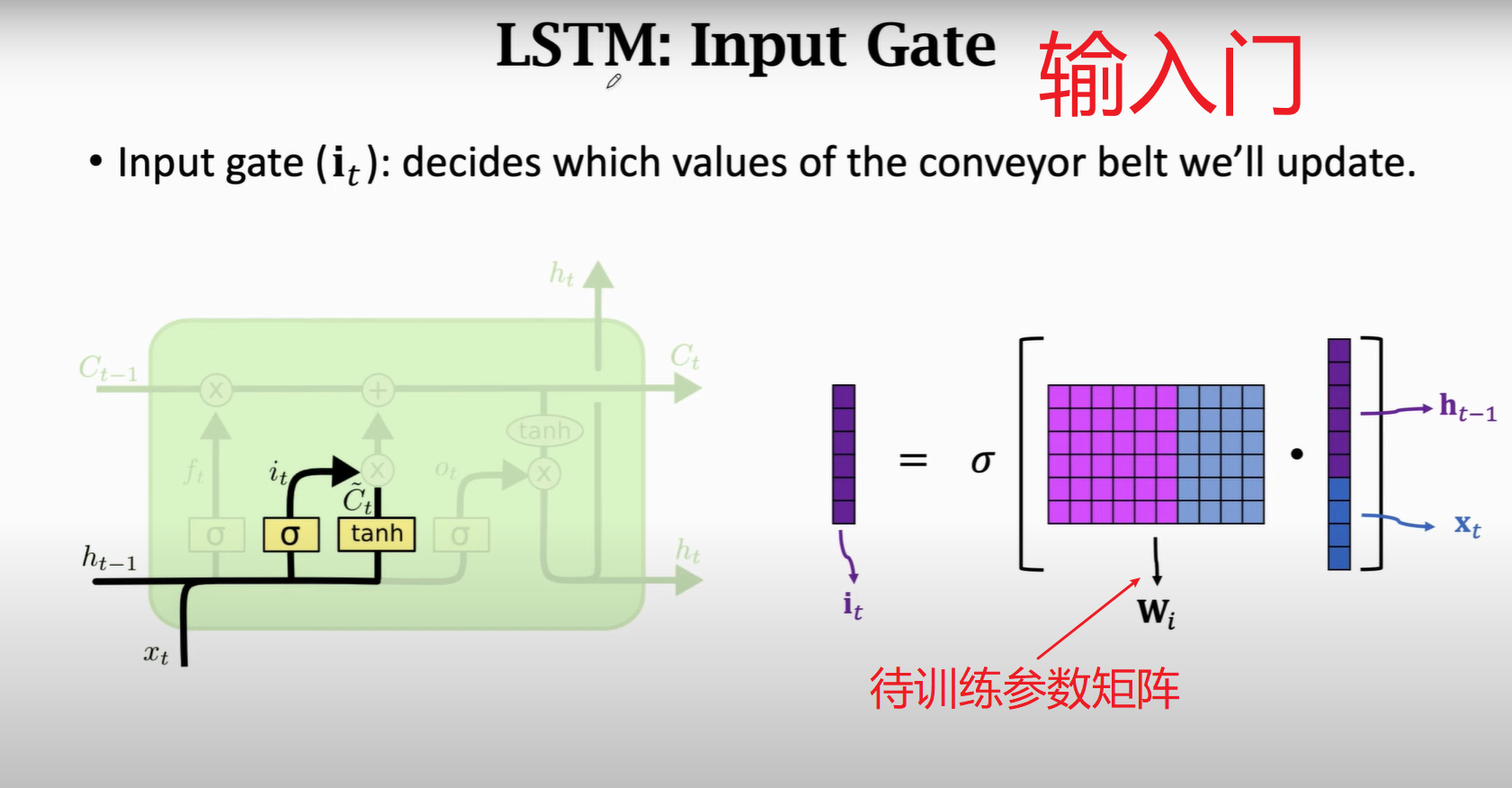
1.3 New Value
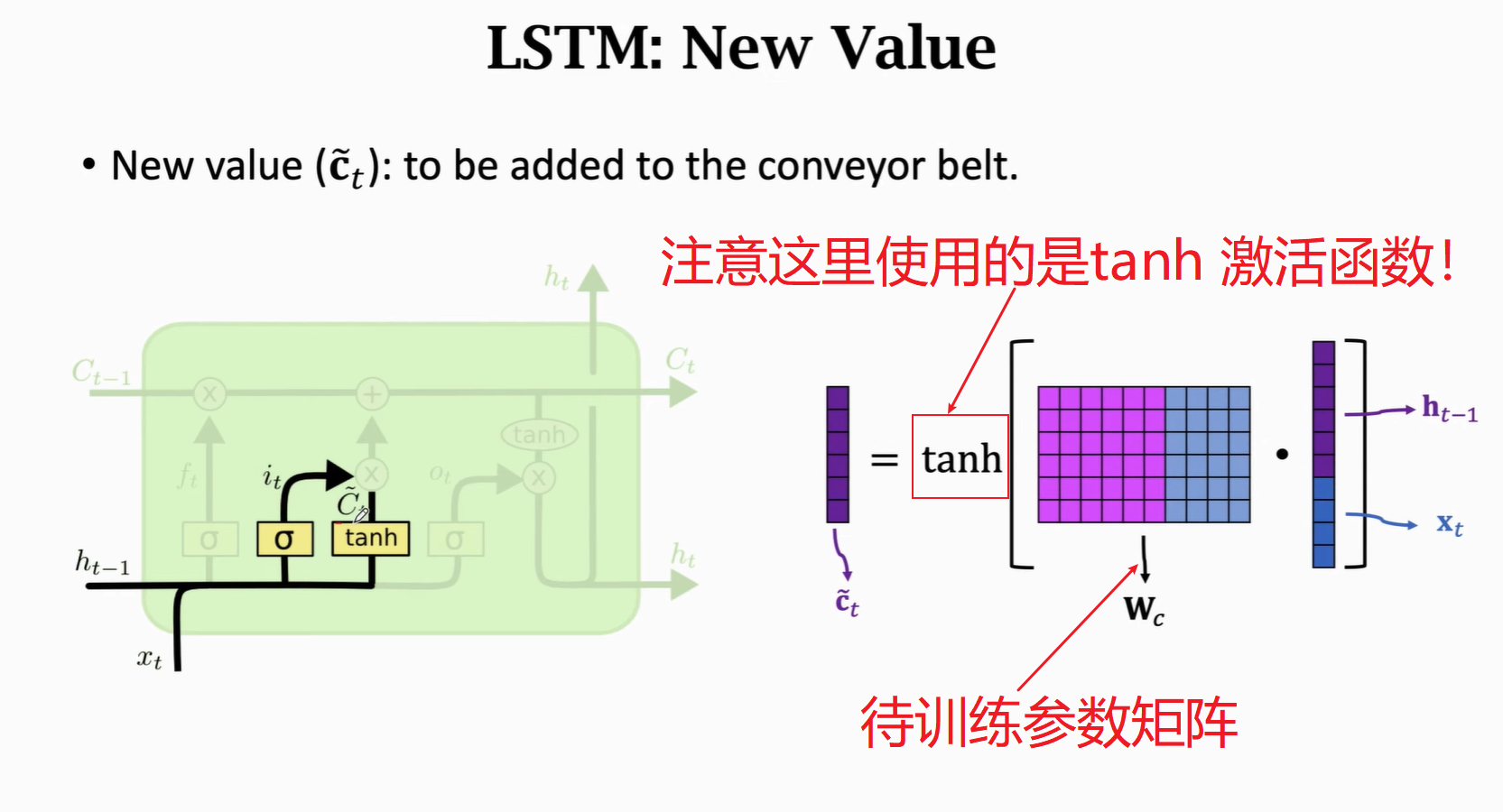
1.4 Output Gate
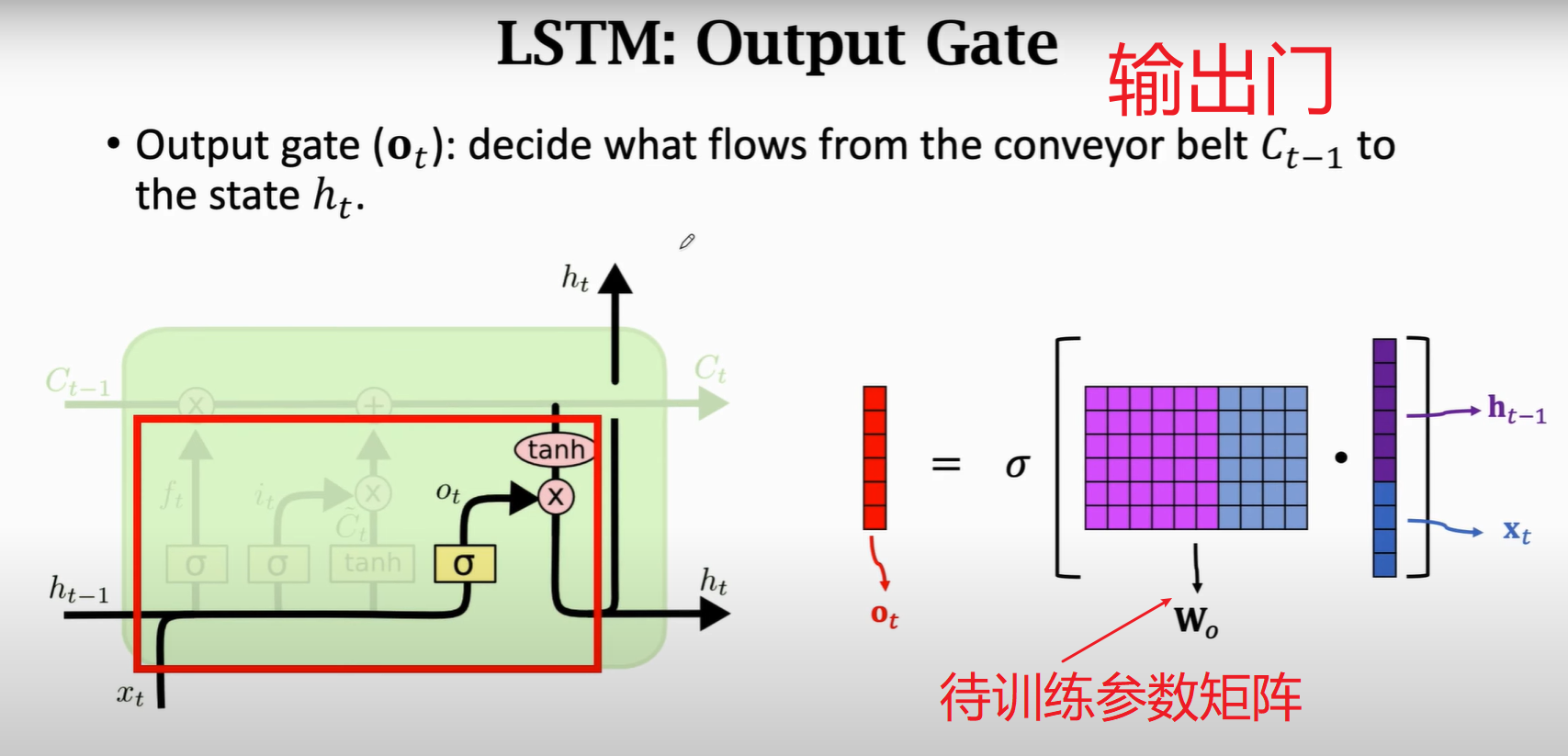
2.Update the Conveyor Belt
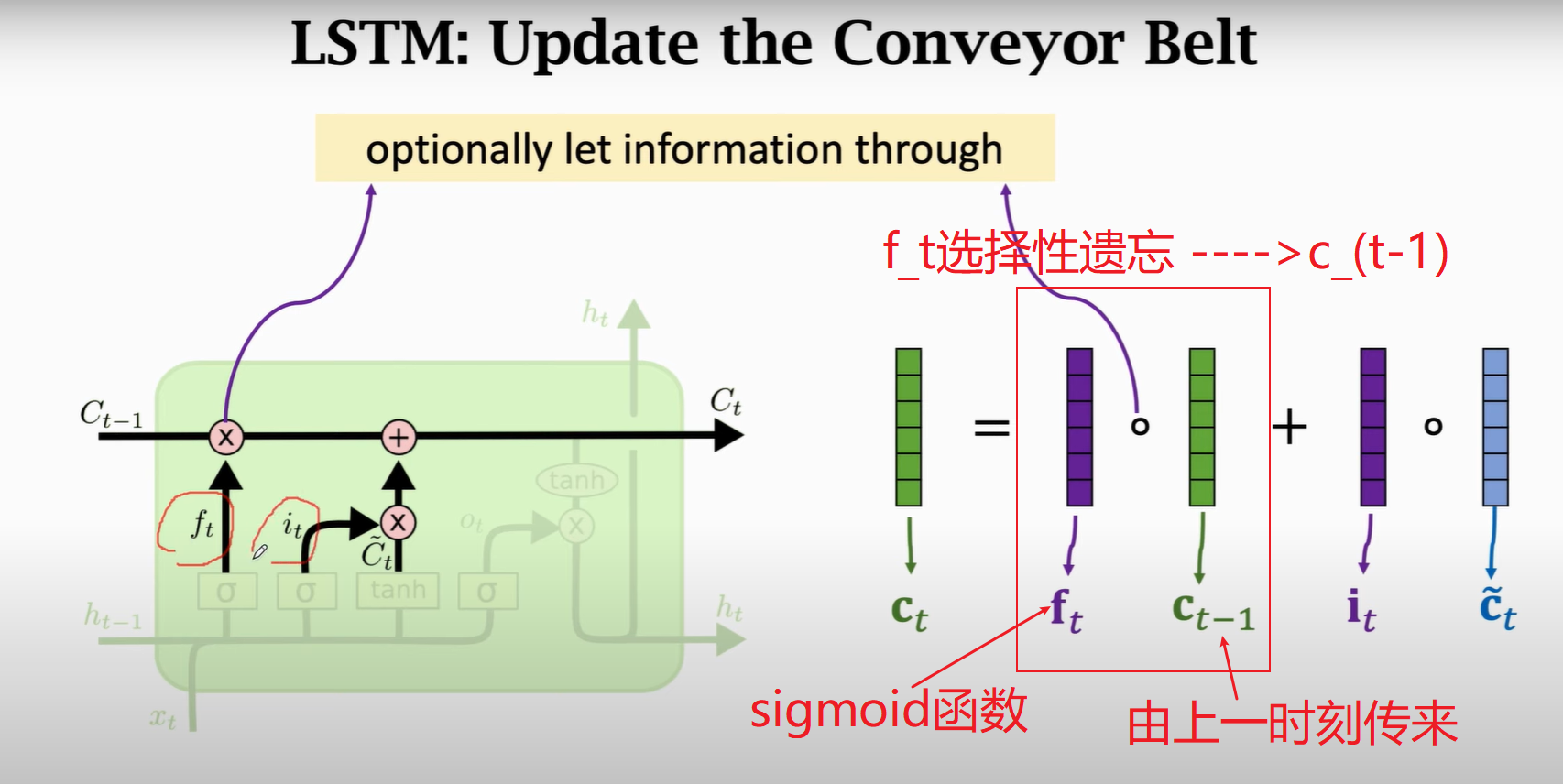
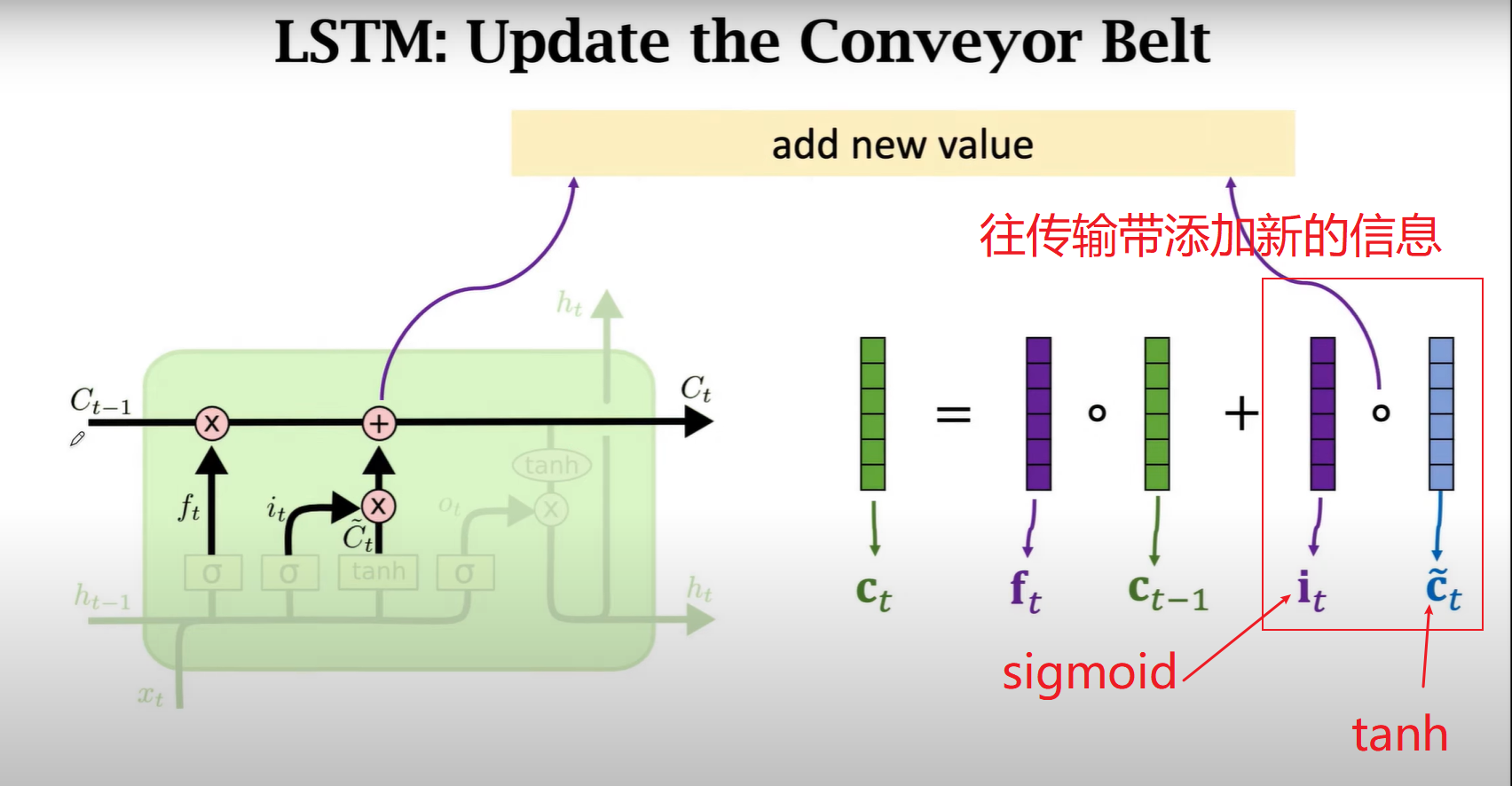
3.Update State
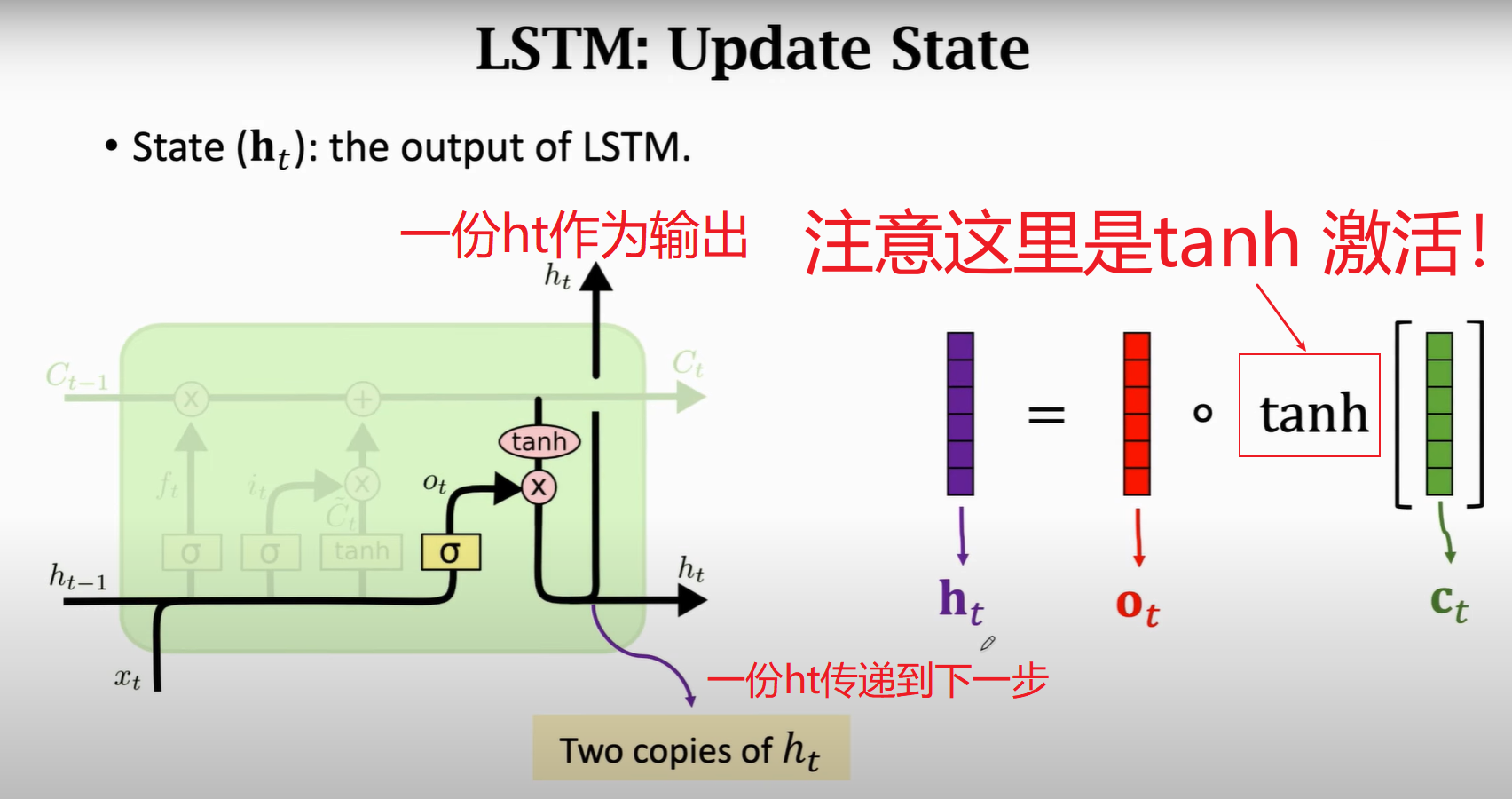
4.Four Matrix
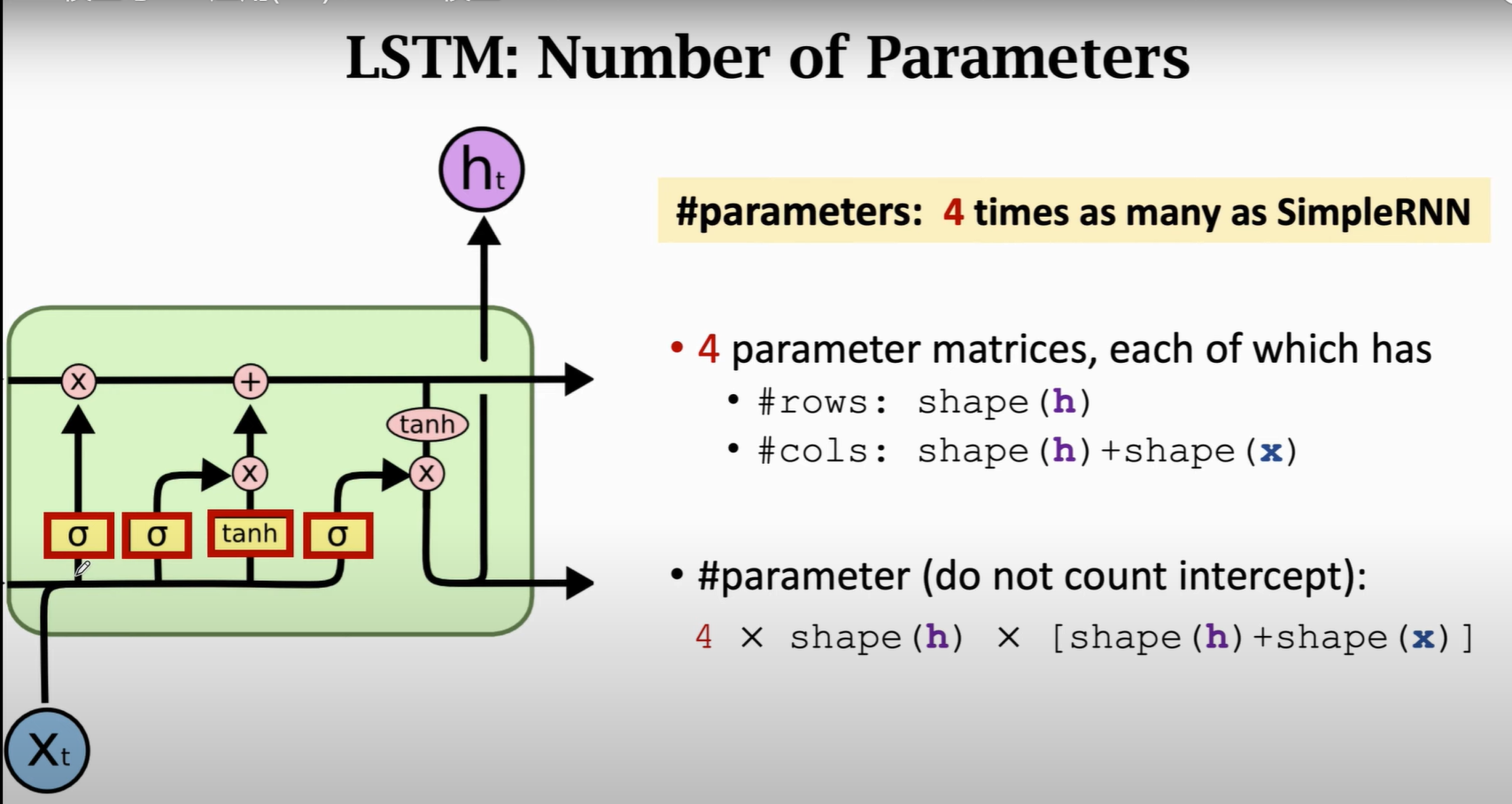
5.Code
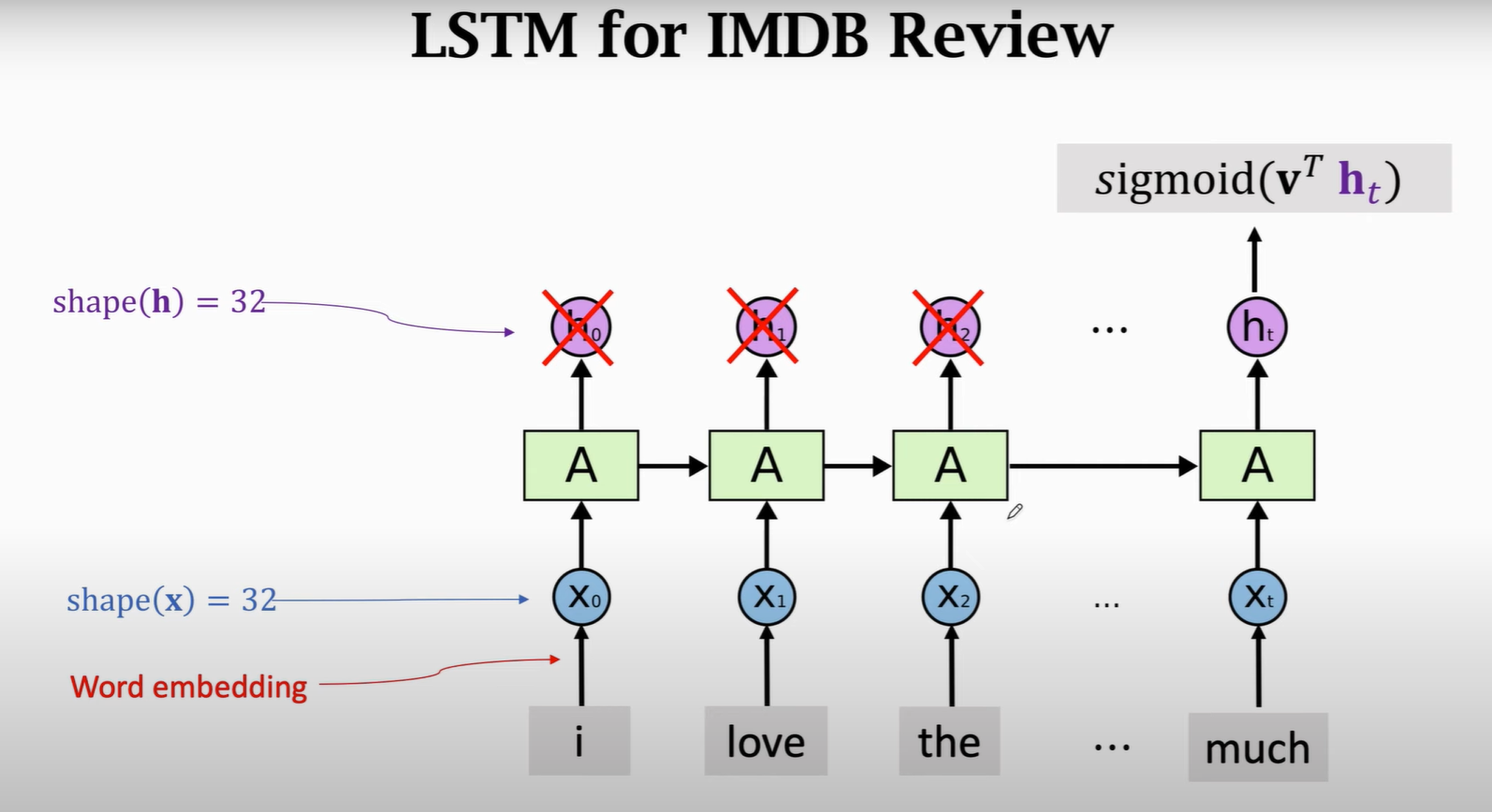
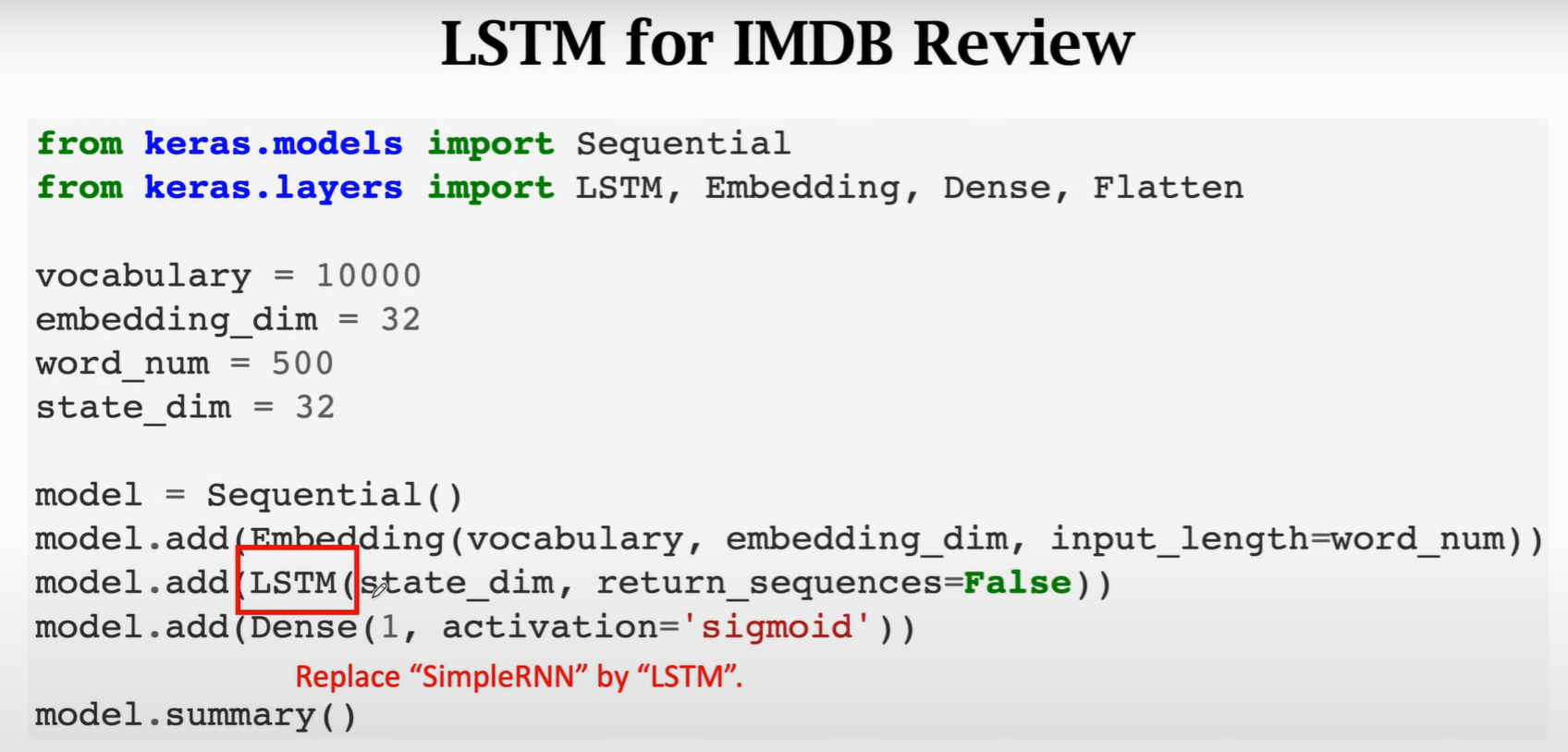
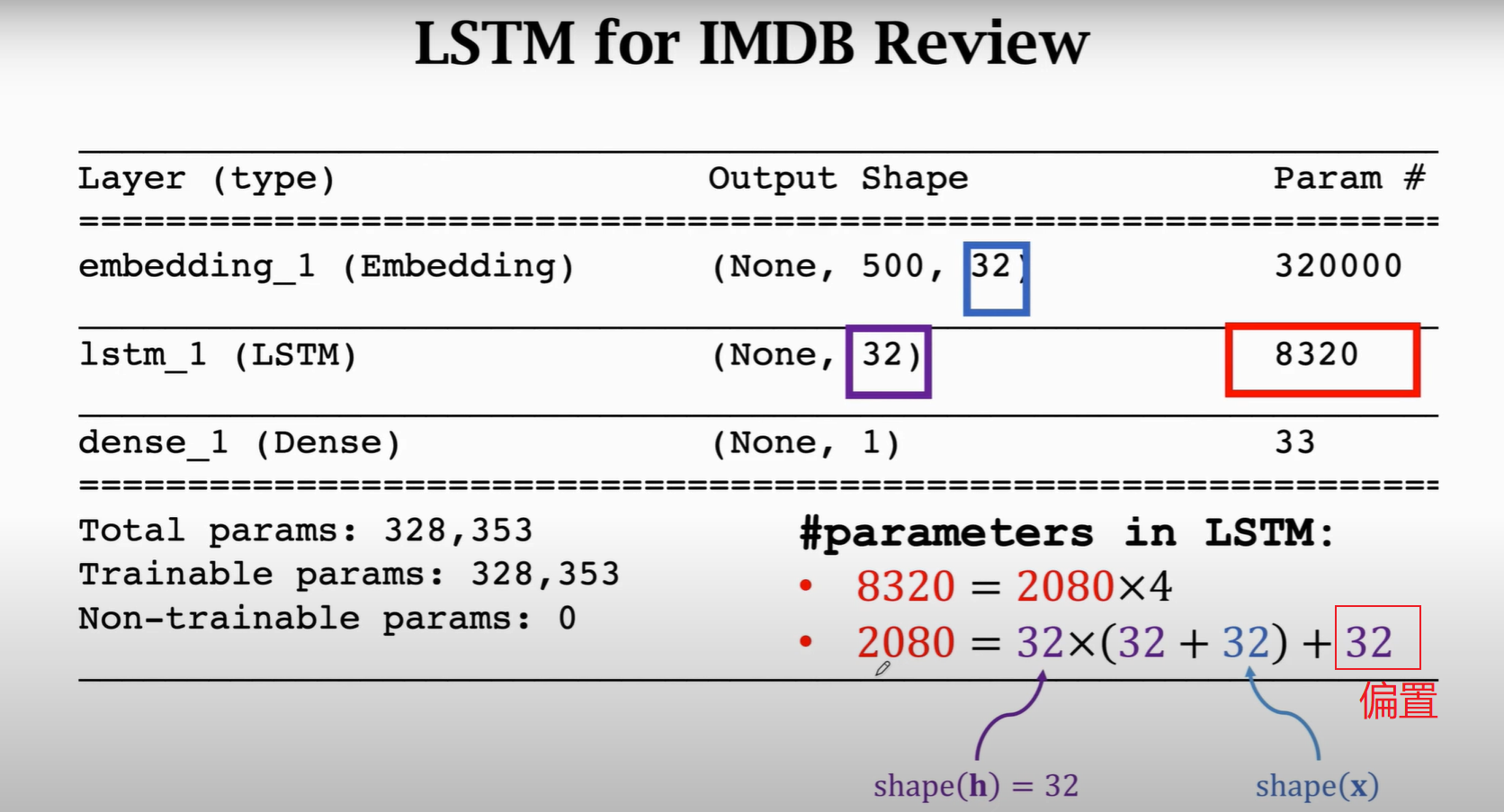
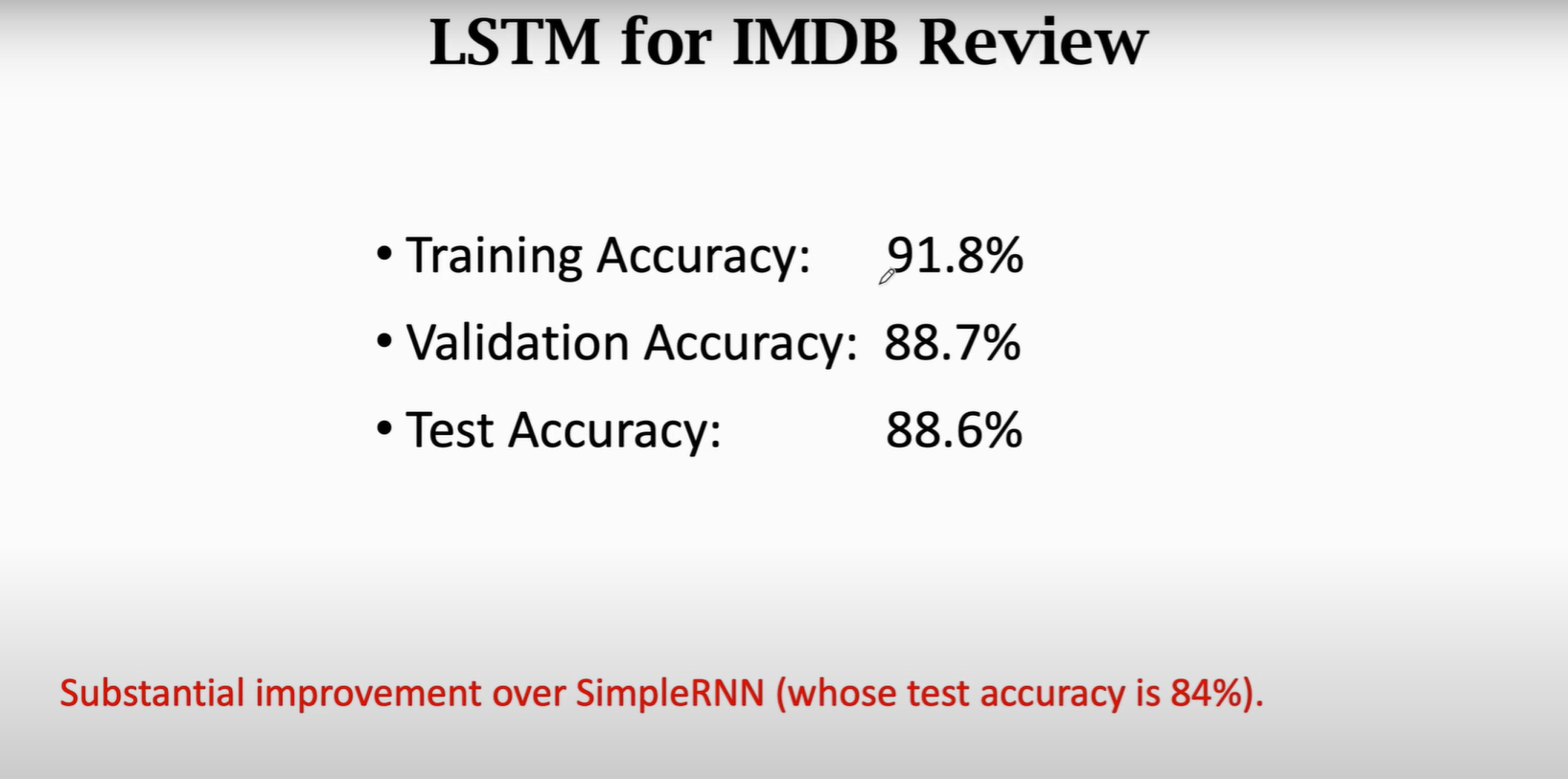
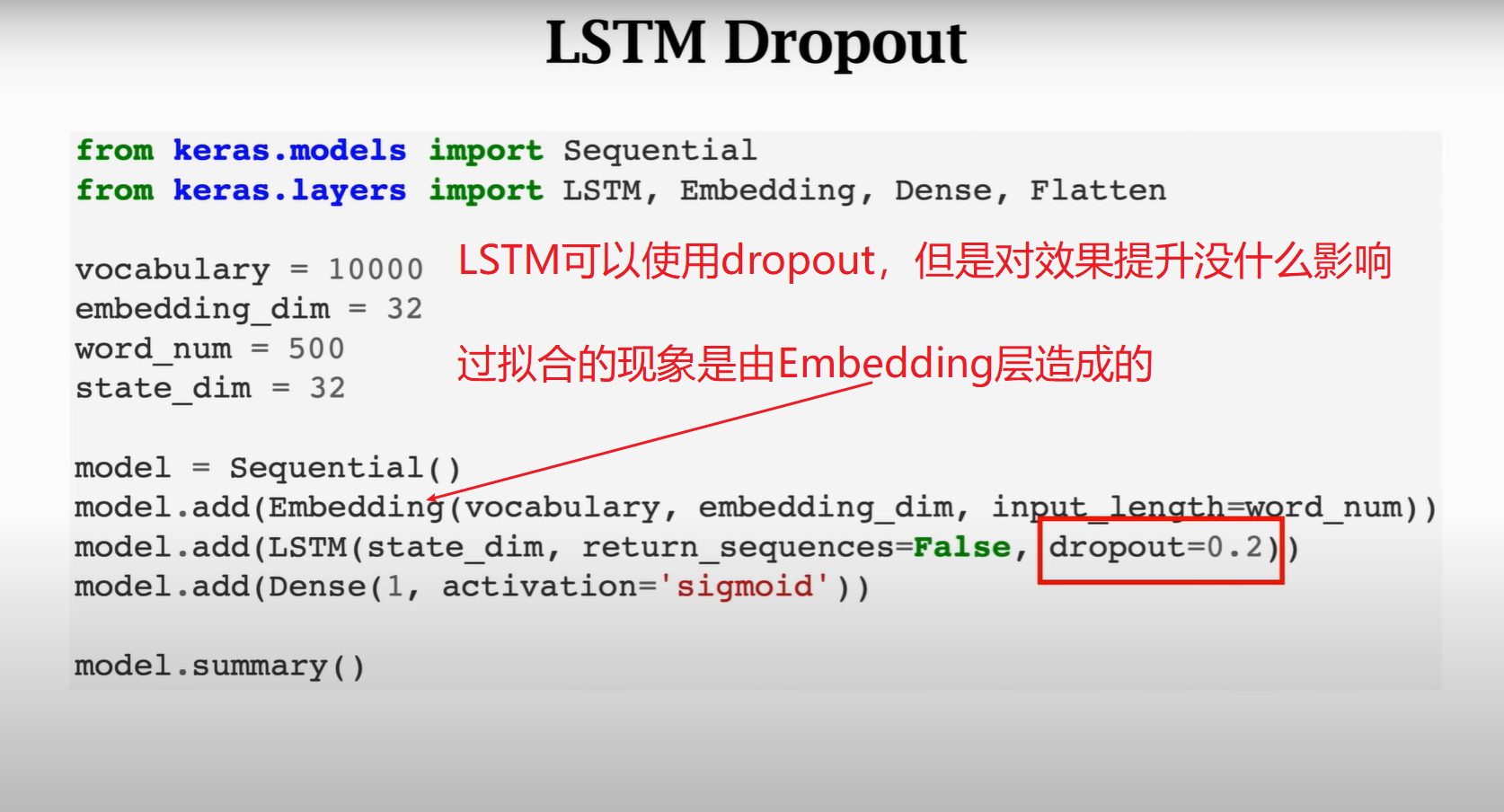
1 2 3 4 5 6 7 8 9 10 11 12 13 14 15 16 17 18 19 20 21 22 23 24 25 26 27 28 29 30 31 32 33 34 35 36 37 38 39 40 41 42 43 44 45 46 47 48 49 50 51 52 53 54 55 56 57 58 59 60 61 62 63 64 65 66 67 68 69 70 71
| import numpy as np from matplotlib import pyplot as plt
import tensorflow as tf from tensorflow.keras.preprocessing import sequence
np.set_printoptions(threshold=np.inf)
epochs = 3 batchsz = 32
vocabulary = 10000 embedding_dim = 32 word_num = 500
state_dim = 32
imdb = tf.keras.datasets.imdb (x_train, y_train), (x_test, y_test) = imdb.load_data(num_words=vocabulary) x_train = sequence.pad_sequences(x_train, maxlen=word_num) x_test = sequence.pad_sequences(x_test, maxlen=word_num)
model = tf.keras.models.Sequential() model.add(tf.keras.layers.Embedding(vocabulary, embedding_dim, input_length=word_num)) model.add(tf.keras.layers.LSTM(state_dim, return_sequences=False, dropout=0.2)) model.add(tf.keras.layers.Dense(1, activation="sigmoid"))
model.summary()
model.compile( optimizer=tf.optimizers.RMSprop(learning_rate=0.001), loss='binary_crossentropy', metrics=['acc'], )
history = model.fit( x_train, y_train, batch_size=batchsz, epochs=epochs, validation_split=0.2 )
acc = history.history['acc'] val_acc = history.history['val_acc']
loss = history.history['loss'] val_loss = history.history['val_loss']
plt.subplot(1, 2, 1) plt.plot(acc, label='Training Accuracy') plt.plot(val_acc, label='Validation Accuracy') plt.ylabel('Accuracy') plt.xlabel('Epoch') plt.title('Training and Validation Accuracy') plt.legend()
plt.subplot(1, 2, 2) plt.plot(loss, label='Training Loss') plt.plot(val_loss, label='Validation Loss') plt.title('Training and Validation Loss') plt.ylabel('Loss') plt.xlabel('Epoch') plt.legend() plt.show()
loss_and_acc = model.evaluate(x_test, y_test) print('on test dataset, loss = ' + str(loss_and_acc[0])) print('on test dataset, acc = ' + str(loss_and_acc[1]))
|
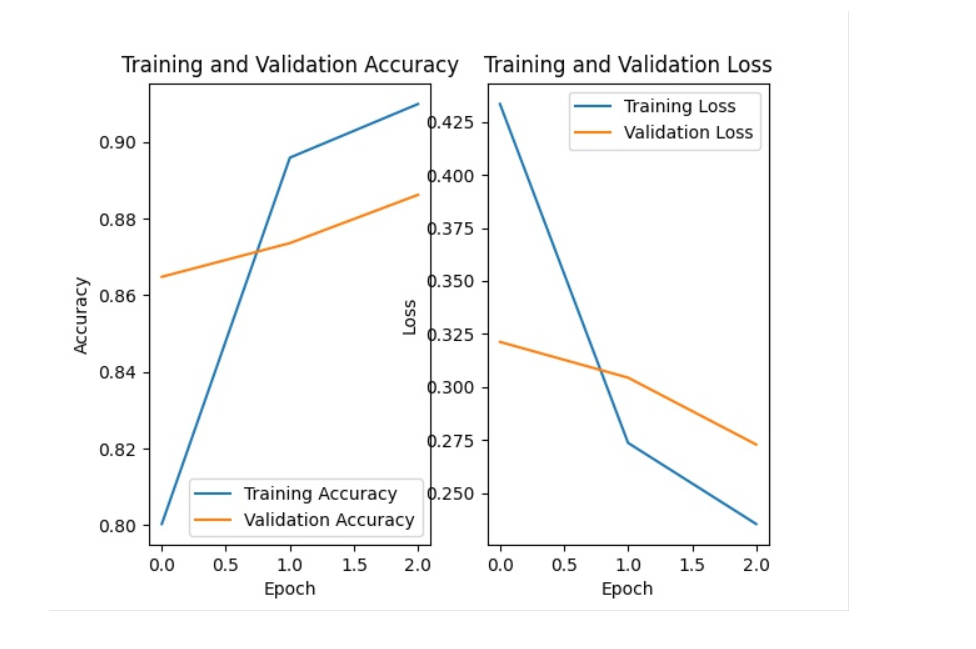
6.Summary
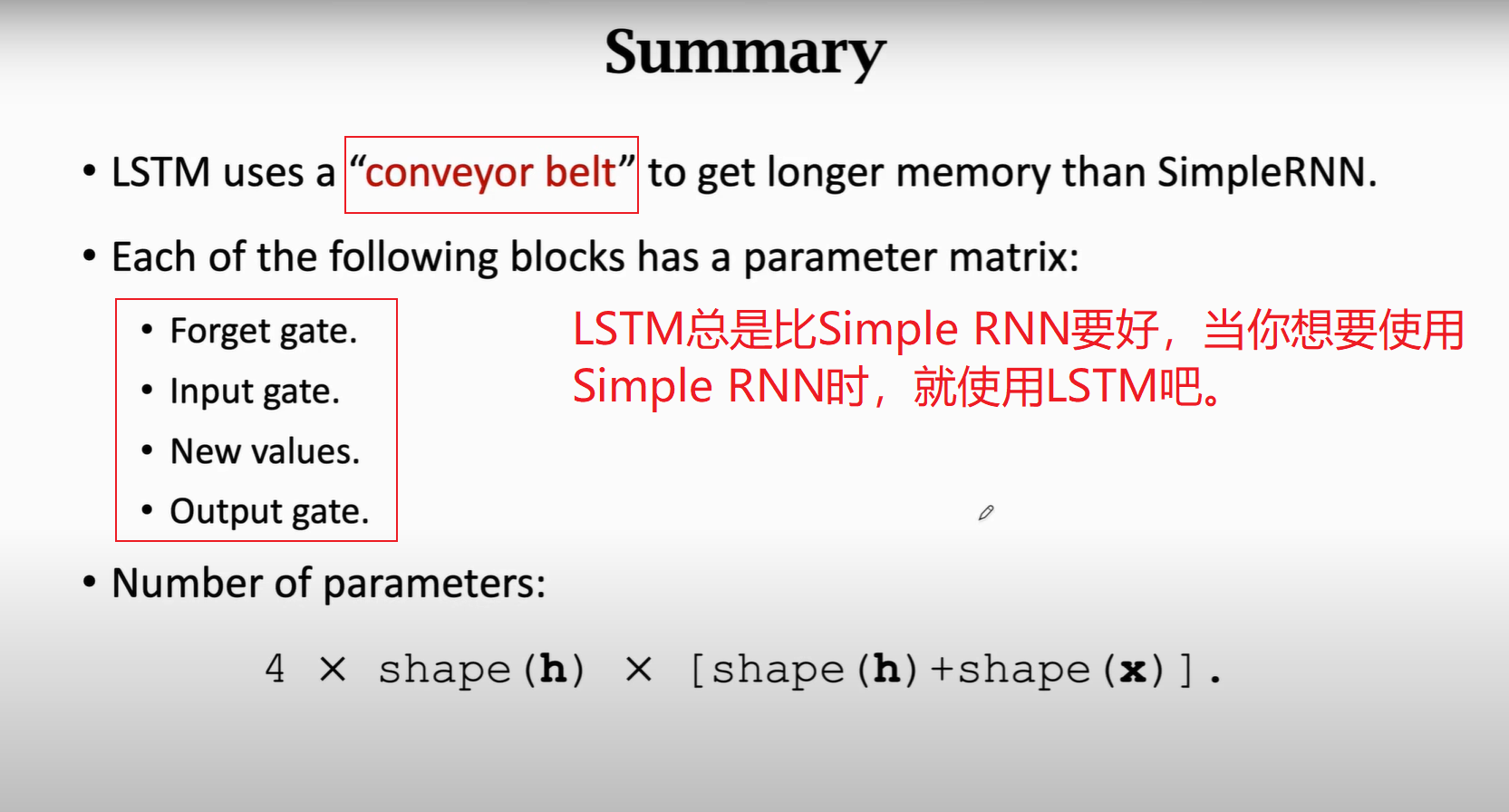